To Issue 160
Citation: Cowen D, White S, Robinson A, “Accelerating Combination Product Development Using Digital Twin Technology”. ONdrugDelivery, Issue 160 (May 2024), pp 16–20.
Dan Cowen, Sebastian White and Anthony Robinson, discuss the value that implementing a digital twin could provide to drug delivery device design across multiple stages of a development programme.
“Digital twin” is a phrase you hear everywhere. Whether it is the enabling technology for Industry 4.0,1 the concept behind next-generation municipal control systems2 or a virtual representation of a human heart used for tailoring personalised therapies,3 the excitement is palpable. However, it is clear that this term is used in a variety of ways, with the possibility for much confusion. So, what is a digital twin and how can it be applied to MedTech, and to drug delivery specifically?
“By leveraging digital twins, engineers and scientists can efficiently iterate design changes, conduct in silico investigations and optimise for key performance indicators.”
WHAT IS A DIGITAL TWIN?
The concept of a digital twin was formalised by John Vickers in 20024 in the context of product lifecycle management. Since then, the idea has been applied to a vast array of industries and sectors.5 At its core, a digital twin is a digital representation of a physical asset, either real or potential, that describes the system in sufficient detail and scope so as to be effectively indistinguishable from reality in the specified use context. Some definitions go further and require that the twin takes data from its real-world counterpart and updates itself in real time. Others go further still, requiring that the digital object is also able to influence the physical object autonomously, so that the two evolve in lockstep under their shared influence and that of the environment.
Categorising Digital Twins
To resolve this apparent conflict of definitions, the nature of the data flow between the physical and digital objects and the degree of integration can be used to classify levels of digital twin representation:
- A digital model is a digital representation of a future product used to prototype in silico within the virtual intended use environment. It is a comprehensive, system level, multiphysics simulation and workflow that is manually informed and validated by a small number of targeted physical test cases and library data sources. It can be used to inform and optimise system designs prior to significant prototype investment.
- A digital shadow is a digital version of an existing physical asset, incorporating the transfer of data from the physical asset to the digital model in near real-time from a network of sensors to enable a deep understanding of the current state of the system and to roleplay changes and “what if” scenarios.
- A digital twin is a digital replica of an existing physical asset. It automates the transfer of data from the physical asset to the digital model; however, in this case, the digital model can instruct the physical asset based on generated insights and scenario explorations to improve the performance of the physical system fully autonomously.
Furthermore, digital twin deployments can be arranged according to the type of physical object they represent:
- A digital twin prototype describes an artefact that does not yet exist. It is therefore entirely predictive and can serve as a blueprint to prototype the physical asset. As there is initially no real-world system to share data with, the digital twin prototype is necessarily a digital model.
- A digital twin instance represents a single, specific physical entity that the twin remains linked to throughout its operational life. An instance may be any level of twin but, given that the entity exists in the real world and is subject to change, it is more likely a digital shadow or a full twin.
- A digital twin aggregate covers a population of digital twin instances and can be used to understand global behaviour and provide insights across the expected range of tolerances and conditions experienced.
These classifications (Figure 1) provide a framework to enable discussion of the types of digital twin out there, although debate still rages about which of these constitute a “true” digital twin.
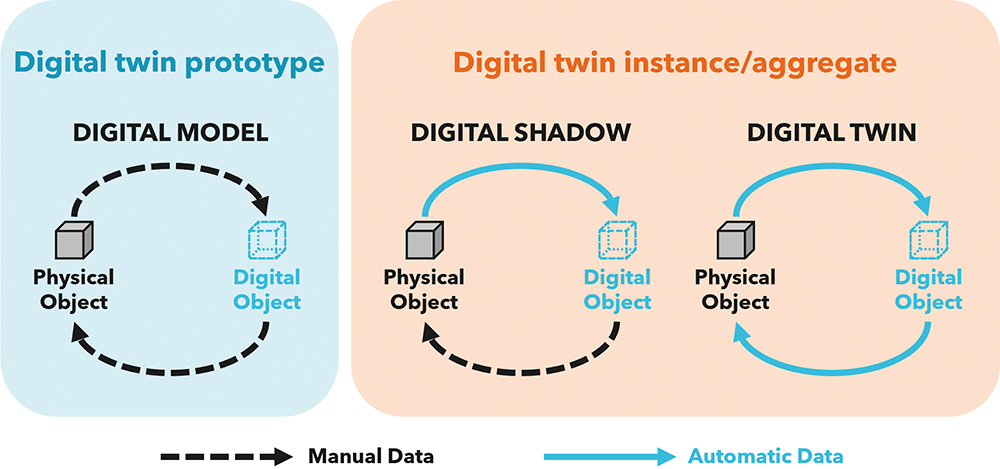
Figure 1: Types of digital twin showing the flow of data between physical and digital assets.
“A digital twin can pay dividends in the range and wealth of data it provides, shortcutting huge chunks of development time and effort.”
DIGITAL TWINS IN DRUG DELIVERY
Digital twins are a great fit for drug delivery systems and could potentially be deployed at any stage in the product lifecycle. As they offer a data-driven approach to the design, manufacture and deployment of these products, they can achieve gains and improvements not possible with traditional methods.
Development
By leveraging digital twins, engineers and scientists can efficiently iterate design changes, conduct in silico investigations and optimise for key performance indicators, all of which generate valuable insights for advancing system designs, with the added bonus of reduced time to market and lower development costs. Digital twin prototypes used in injectable product development are explored later in this article. Digital twins also offer an amazing opportunity to accelerate drug discovery, simulating the interaction between candidate molecules and the human body to better understand the therapeutic benefit and potential side effects, as well as to personalise dosage and administration frequency.6 Major players in the pharma industry are currently partnering with digital service providers to develop twins for this application.
Manufacturing
Beyond the design phase, digital twins offer significant benefits in manufacturing processes. From optimising facility layouts to ensuring operational efficiency, digital twins deployed in the manufacturing environment can increase efficiency, minimise downtime, improve quality and, ultimately, reduce costs.7 They are a key enabling technology behind the shift to smart factories and intelligent industry.
Deployment
In addition to supplementing the design process and optimising manufacture, digital twins can expand and improve current drug delivery device use cases. With their ability to simulate real-world scenarios and predict performance outcomes, digital twins can, via continuous monitoring, ensure that deployed devices are performing as intended and dynamically make necessary set-up changes if they are not.
One nearer-term possibility might be next-generation control algorithms for electromechanical autoinjectors that use an internal digital representation of themselves to update operating parameters for greater performance and to deal with novel use conditions. Similarly, such continuous monitoring can also provide medical practitioners with valuable data regarding device usage and patient health to supplement their decision-making process.
A possible future sees drug delivery device digital twins integrated with patient digital twins to optimise therapies digitally and continuously on a case-by-case, day-by-day basis. Of course, much of this is very future-looking and there are significant technical and regulatory hurdles to overcome, similar to the use of artificial intelligence (AI) in healthcare settings, but the potential reward and improvement to patient outcomes is enormous. Full digital twins have the capacity to change how we perceive and interact with drug delivery systems and, ultimately, transform how patients benefit from them.
DIGITAL TWINS SUPPORTING PRODUCT DEVELOPMENT
In the near term, digital twins have the potential to transform the development process for drug delivery systems, reducing development timelines and costs while simultaneously improving development outcomes. Digital twin prototypes can be constructed to assess candidate products and system designs digitally in a virtual use environment long before anything is physically made and tested.
This in silico representation of the proposed system enables rapid iteration and optimisation of designs across an extensive design space, giving greater access to system parameters, conditions, levels of variation and sheer number of test points than could feasibly be realised in a laboratory environment. The simulated product also gives access to a world of data that would be difficult or even impossible to generate physically, which can be used to gain a deeper understanding of the system behaviour. Once this process of iteration and optimisation against stated goals delivers a satisfactory design, the digital twin serves as a blueprint for physical realisation, with parts prototyped and assembled for testing to ensure that performance is adequate and to assess empirical results against the in silico twin predictions.
This holistic system view offers a step change in product development approach, allowing multiple trade-offs and performance optimisations to be conducted simultaneously, using advanced optimisation routines and AI-enabled development methods. This differentiates digital-twin-led design from simulation-led design (Figure 2), where discrete one-off simulations of specific physical domains are used to inform engineering insight. However, simplifications and assumptions using idealised boundary conditions can lead to errors, and integration of many individual domains may not adequately represent the entire assembled system.
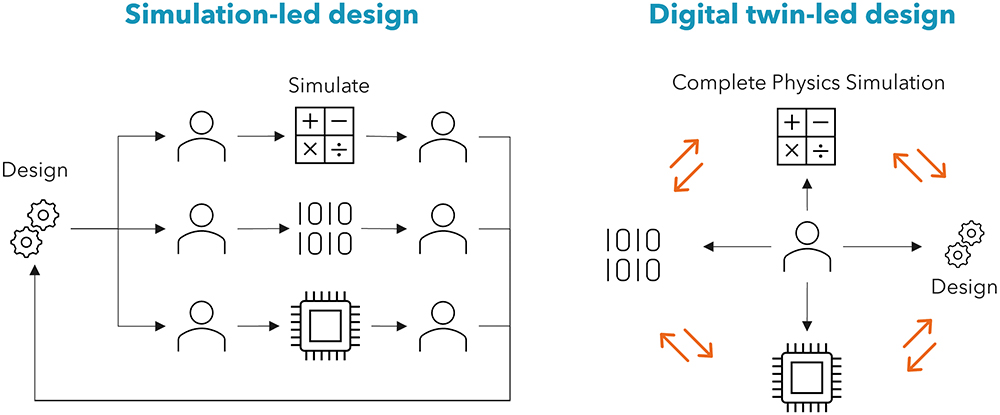
Figure 2: Simulation-led vs digital-twin-led design.
Use of a digital twin prototype during early development can be particularly beneficial. It is estimated that 80% of the product performance (including cost and sustainability) is baked in during the design phase8 – digital twin prototypes allow us to deliver the most meaningful impact at the most impactful time. They also present the opportunity to reduce development costs and timelines, allowing ideas to go from product brief to clinical trial faster, cheaper and with greater confidence than ever before.
Considering their use in injectable device development, the deployment of a digital twin prototype enables the prediction of key aspects of component, sub-assembly and system performance as a linked computer-assisted design model is detailed and built up, shortening development timelines and saving physical iterations, but the power also extends far beyond this.
Imagine specifying the ideal motor to perform a set of functions in an electromechanical autoinjector, then substituting in parameters from a real motor specification selected based on these performance requirements to ensure satisfactory function. Imagine predicting the use life and charging cycle of the battery, based on the full system performance across a range of likely use scenarios and automatically finding an appropriate part for the bill of materials. Imagine defining a loss function to optimise the entire design for formulation delivery performance, cost and sustainability simultaneously.
By way of example, consider specifying an injector spring for delivering the formulation from a drug container through a needle. Traditionally, simply understanding the expected injection force would involve extensive hand calculations, some level of modelling and an assortment of time-consuming lab tests that are probably not even an ideal substitute for testing in living human skin. With a digital twin prototype, the system can be represented in silico, including the effects of manufacturing tolerances, environmental conditions and so on, then combined with a virtual skin model that can represent a range of patients of different ages, genders and ethnicities, as well as a variety of injection locations. This input space can be explored efficiently to give an estimate of performance over a broad operating envelope, allowing the specification of a suitable component with the confidence that it can handle the full range of expected conditions.
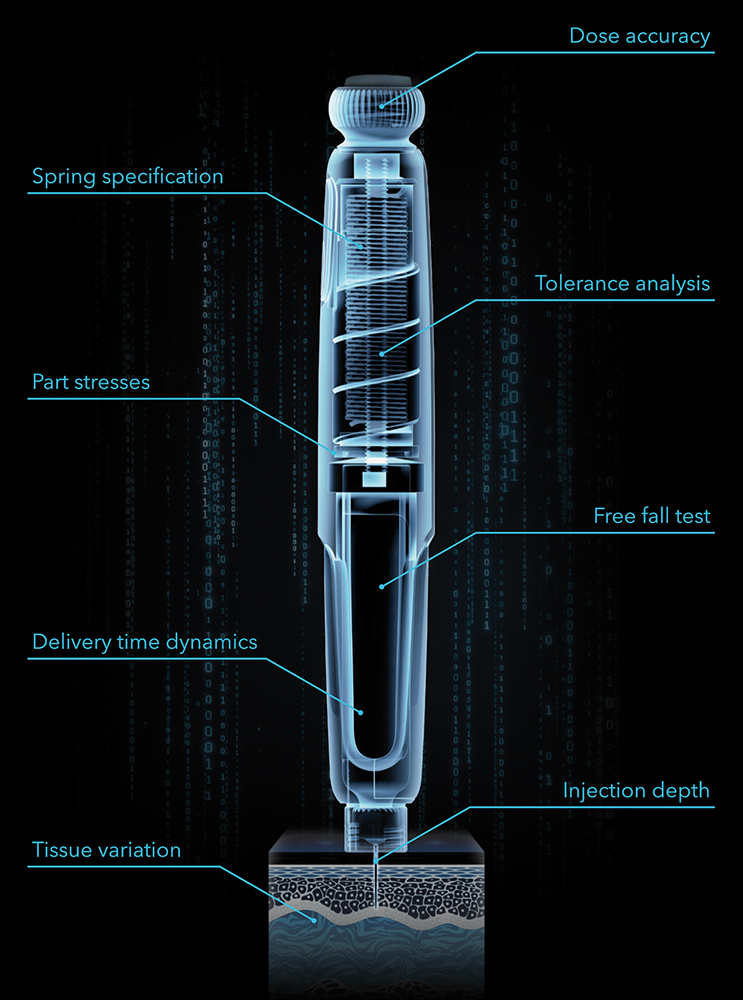
Figure 3: Examples of development tests and optimisations that can be performed in silico by a digital twin prototype.
Many standard needle injection system tests demanded by ISO 116089,10 require full devices to be prototyped and tested, which only happens late in the development process. The free fall test, for example, requires the developer to design an expensive and complicated injector system according to best practice, splitting it into subsystems where great effort is put into each specific area, with hand calculations, single physics simulations and a good measure of engineering experience and judgement. Then tens or even hundreds of parts are painstakingly prototyped at great monetary cost, involving injection mould tools, printed circuit board assemblies, custom springs, etc, before the prototyped design is finally built; at which point it is dropped, it breaks and the test is over – back to the drawing board. If instead, the developer built a digital twin prototype early in development and subjected it to a battery of virtual tests – drop tests, storage conditions, dose accuracy, needle insertion, depth control, injection time, etc (Figure 3), they would have the potential to pre-empt the drop test result and design around it before any parts have been made in the real world. It is possible to pore over the results, performing failure analysis and interrogating part stresses and transient evolution of parameters, and iterate to a robust and reliable design that takes most of the risk out of later physical testing.
There’s no denying that setup and deployment of a digital twin is challenging, with a significant upfront investment of time and effort to define and implement the twin, possibly requiring custom data inputs and targeted characterisation experiments, all of which need to be of adequate accuracy and fidelity, but, once this has been completed, a digital twin can pay dividends in the range and wealth of data it provides, shortcutting huge chunks of development time and effort. Once a particular application is well-understood, the developed assets, such as tissue models or electronic component representations, can be redeployed on future development programmes, expediting them even further. Investment and experience are required to make a success of this, but the potential payback is high (Table 1).
Advantages | Challenges |
|
|
Table 1: Advantages and challenges of implementing a digital twin prototype.
CONCLUSION
Digital twins offer an amazing opportunity to transform drug delivery devices during development, production and deployment. Digital twins are gaining traction in injectables manufacturing where they can be deployed to minimise losses and improve production efficiency, and the time is right to implement them during the development stage too. This promises to maximise performance gains by designing the right thing first time, all while reducing development timelines, development costs and development risk.
REFERENCES
- Frank D, “Digital-Twin Project Green-Lights Traffic Congestion Improvements”. NREL, Jun 8, 2023.
- “How Digital Twin technology can enhance Aviation”. Company Web Page, Rolls Royce, Accessed Apr 2024.
- “The Living Heart Project”. Web Page, Dassault Systèmes, Accessed Apr 2024.
- Grieves M, Vickers J, “Digital Twin: Mitigating Unpredictable, Undesirable Emergent Behavior in Complex Systems” in “Transdisciplinary Perspectives on Complex Systems” (Kahlen F-J, Flumerfelt S, Alves A Eds). Springer, 2017, pp 85–113.
- “Digital Twins: Adding Intelligenceto the Real World”. Report, Capgemini, 2022.
- An G, Cockrell C, “Drug Development Digital Twins for Drug Discovery, Testing and Repurposing: A Schema for Requirements and Development”. Front Syst Biol, 2022, Vol 2, Article 928387.
- Badiei A, “Digital Twin in the Pharmaceutical Supply Chain.” ONdrugDelivery, Issue 149 (Jun 2023), pp 45–58.
- “Circular economy action plan”. Web Page, European Commission, Accessed Apr 2024.
- “ISO 11608-1:2022 Needle-based injection systems for medical use: Requirements and test methods Part 1: Needle-based injection systems”. International Organization for Standardization, Apr 2022.
- “ISO 11608-5:2022 Needle-based injection systems for medical use: Requirements and test methods Part 5: Automated functions”. International Organization for Standardization, Apr 2022.